Marcus Olivecrona, our Lead Machine Learning Engineer, walks us through AI
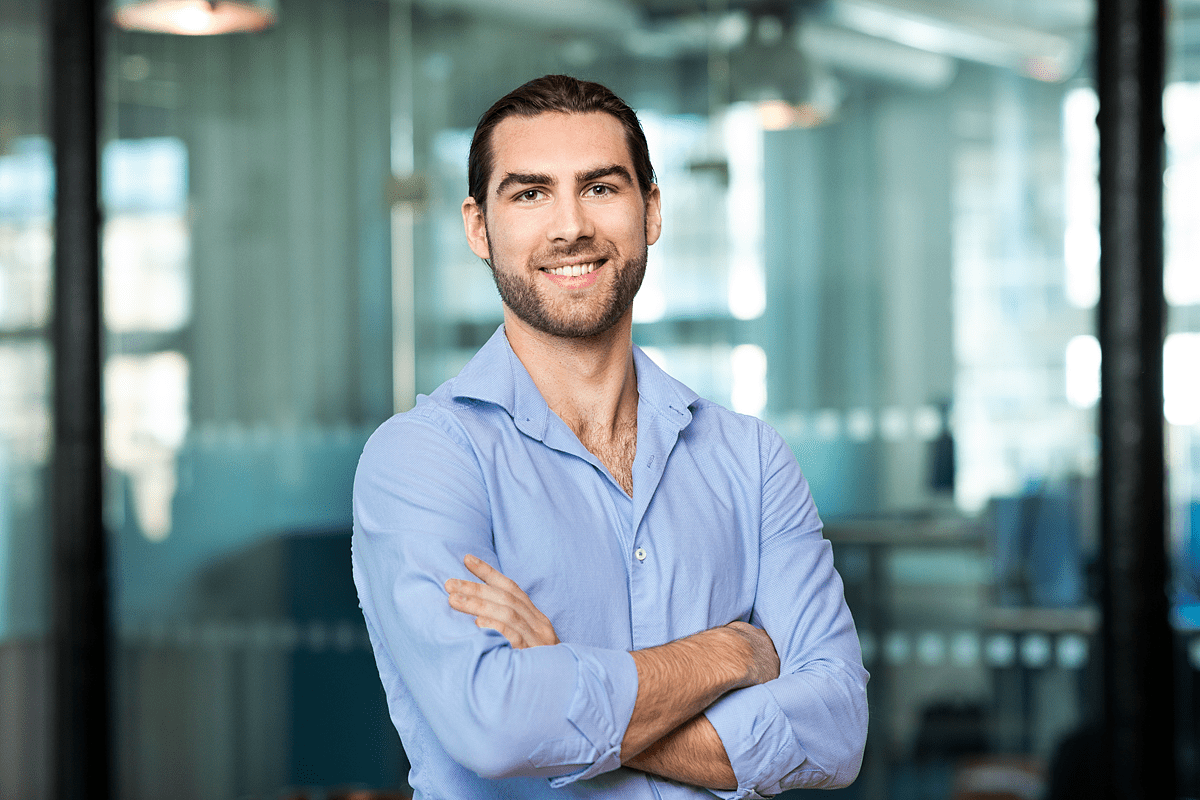
Marcus Olivecrona has been a decisive driver behind Red Robin, Visiba's symptom assessment tool, since its conception. This autumn, he joined Team Visiba full time as a Lead Machine Learning Engineer and we are beyond thrilled to have him on board to continue making Red Robin a valuable tool for primary care. In this interview, Marcus demystifies the term AI and places this technology in the context of healthcare, an area he has been in contact with his entire life.
Tell us a bit about your background
I'm a chemist by training; I did my Bachelor’s and Master’s at Oxford and then I went on to a graduate program at AstraZeneca, which was rather multi-functional. I slowly transitioned from doing synthetic chemistry, which is what I did during my Master’s to machine learning and AI, which is what I'm doing right now. After that, I started working as a machine learning engineer at Tenfifty, here in Gothenburg, where I started consulting on the Red Robin project, and now I’m at Visiba full time!
Was it life that brought you to machine learning or was it something you actively went after?
It was a bit of both. I have always been interested in programming; it's always been something I wanted to do, but I never really got around to doing it and there wasn't any clear opportunity before AstraZeneca. Once there was an opportunity, I grabbed it and I felt that I found myself: My strengths as a person really aligned with the skills that are helpful as a machine learning engineer, whereas my strengths perhaps didn't quite align with what it takes to be a synthetic chemist!
What made you turn from collaborating with Visiba on a consultant basis to getting here full time?
I feel a strong connection to healthcare. It's a big part of my family, everyone is a doctor, and when you're 15, you want to do everything except that. Now 10-15 years later, I think slightly differently so I really like working within healthcare; it feels like this is a position where what we are doing can make a difference in society. That was the strongest selling point for me.
Can you tell us a bit or about Red Robin, the product you will be working on?
Red Robin is a symptom assessment tool. The patient fills in some symptoms and Red Robin suggests which diagnoses –if any– might be causing these symptoms. We use a Bayesian network, a probabilistic causal model, where you describe how different conditions affect each other. To exemplify, your age and lifestyle might affect the risk of getting pneumonia and pneumonia as a condition affects your probability of having a cough. The basis is medical knowledge and figuring out the kind of questions that are the best to discern between possible diagnoses can be quite tricky sometimes. We occasionally use data points which are not based on patient input, such as image analysis to analyse pictures of the skin and incorporate this with the other responses from the patient.
However, Red Robin mostly asks the patient about their symptoms. For some things, it's easy to answer as a patient, but for other things it might be harder. This assessment is traditionally done by an experienced clinician, and it can be challenging to figure out how to question a patient about certain symptoms in order to reach the same degree of precision as the clinician, but we try to come as far as possible!
What is the biggest misconception about AI in your experience?
I think it depends on who you talk to, but I would say the fact that it is called intelligence. That is perhaps a philosophical question – ‘what is intelligence?’ – but the kind of AI we have today cannot reason; it does not have common sense. On this ground, I think statistical learning is perhaps a better term than AI, which can bring the connotation of an all-knowing entity that can evolve. That is a state where we might get in the future but most experts would say we are nowhere near – we are so far away that we don't even know how far away we are! My belief aligns with those.
Right now, AI can become very good at very specific things. At a specific task in most domains, an AI system can challenge a human but it does not have the ability to think outside the box. For example, if you see a doctor and your issues are uncommon or are not presented in a typical way, the doctor may be able to figure out a new way of presenting the questions or assessing you, whereas an AI system would be constrained to the kind of data points built into the system – it cannot expand outside of this.
Where do you see AI having the biggest impact on healthcare?
The biggest impact I think is the increased availability of healthcare and monitoring. If I think about Red Robin, anyone can have access to symptom assessment at any time. Additionally, if you are at the hospital, you could have monitoring systems following your condition – or even at home with a smartwatch, you can look for indicators of poor health. Before that, you would visit healthcare when you are in a really bad condition and now you might be able to detect this much earlier. I believe having this kind of personal healthcare available at all times will have a huge impact!
How far are we from an AI system becoming just another tool and what are the influencing factors?
I think we're pretty close! There's always an adjustment period, both for patients and healthcare professional but I don't think this period is actually very long. Now with the pandemic, healthcare did not have many options aside from moving to the digital space. When something is new, there are always some issues too to work out but overall it's going really well and I see the same thing happening here. I think that, as long as there are good, user-friendly systems available out there, they will be used and it will feel natural.
Why is it important that users know that a system is developed on AI?
It can be important in terms of knowing what to expect: What are the strengths and weaknesses of this system, but overall, AI is just another way of solving problems. If you can build a functional system that performs its job, it doesn't matter which technology is used. In this case, it just so happens that AI is the right choice of technology, but it shouldn't matter for the patient: They should use Red Robin and feel like this takes care of their problem.
What strategies can be used to ensure that an AI system is as unbiased as it can humanly be?
This is a big challenge and a difficult problem – it is something to consider when it comes to conducting studies in general. If you are aware of some variable that you want to control against, you can do it, but if you are aware of some variable that you suspect needs to be controlled against, it is fairly straightforward to do, but it does require this awareness. For example, men have more heart attacks and smoking can cause heart attacks; Men have been smoking more than women, at least historically. Is it that men independently of their smoking have a higher risk of heart attack or not? In this case, we know the answer but in other cases, we might not so it presents a real challenge and it is something everyone involved should be aware of – especially in cases of end-to-end trained systems, where there is not always a way to adjust for this: the data input will determine the kind of model that will be used. If you have the kind of correlations that Red Robin is using, for example, I think it's not a difficult a problem to deal with but it is definitely something to be aware of and careful about and we are constantly paying attention to.
What do you do when you are not working?
I do a good amount of sports: A little bit of running, weight training. I play music: guitar and singing. I also like to cook. Nowadays it's home-brewed beer and sourdough pizzas on the menu!